The artificial intelligence (AI) market is undergoing a monumental transformation fueled by exponential advancements in hardware, data centers, software, and the growing demand for scalable AI inference systems. While headlines have fixated on GPU dominance by Nvidia (NVDA) there is far more beneath the surface. Infrastructure investments continue to outpace AI application revenue, yet this imbalance mirrors historical cycles seen with cloud computing and the early internet. By exploring compute, networking, storage, and emerging edge inference, investors can identify areas that have yet to play out and fully position themselves ahead of the curve.
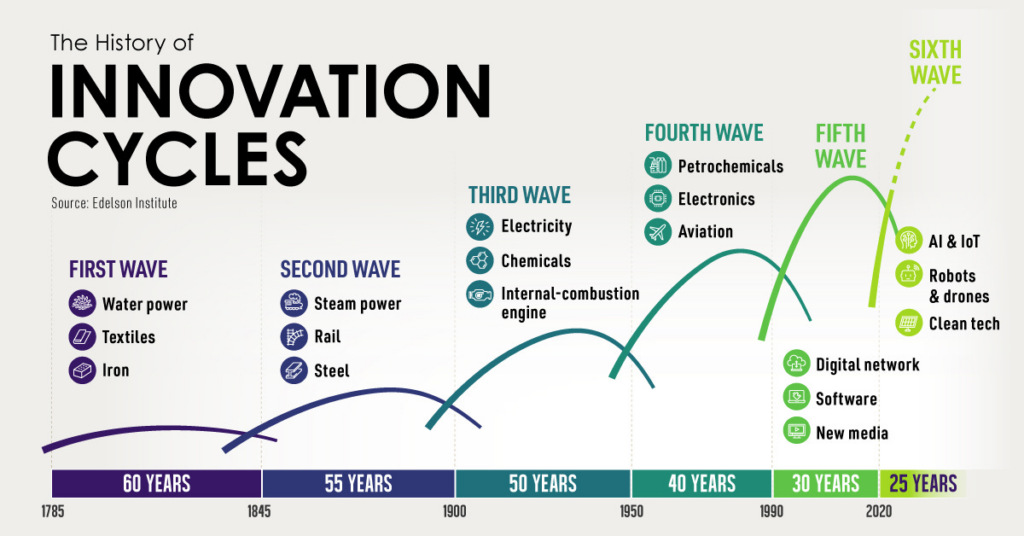
Big Tech’s $275 Billion AI Bet: The Race to Monetize the Next Digital Revolution
An “impressive” surge in CapEx from the “Magnificent 7” of technology giants-Apple (AAPL), Microsoft (MSFT), Amazon (AMZN), Alphabet (GOOG), Tesla (TSLA), Nvidia, and Meta Platforms (META) -adds to the sense that the tech giants are accelerating their efforts to develop or promote full end AI capabilities. Big Tech’s AI CapEx is surging at an unprecedented rate, with over $240 billion expected to be spent in 2024, up 56% year-over-year, and projected to exceed $275 billion in 2025.
The investments are focused on three key areas: data center expansion, where new global facilities are being built to support compute-intensive AI workloads; GPU procurement and custom silicon, with Nvidia’s H100 GPUs in particularly high demand alongside the development of proprietary accelerators like Google’s TPUs and Meta’s MTIA chips; and AI R&D and software, as companies integrate generative AI into cloud platforms, productivity tools, and consumer-facing applications. This aggressive spending reflects Big Tech’s confidence in AI’s transformative potential, with IDC estimating AI’s global economic impact at $20 trillion by 2030. Early signs of ROI are already evident, with Microsoft achieving a $10 billion annualized AI revenue run rate and Amazon’s AWS AI business growing at a triple-digit pace.
For investors, the opportunity lies in monitoring tangible returns as AI products and services scale. Near-term indicators of ROI include growing AI-driven revenue streams (e.g., Microsoft’s Copilot suite and Azure AI APIs), increased adoption of generative AI by enterprises, and improvements in operational efficiency across Big Tech. While Nvidia remains the dominant GPU supplier, other areas such as data center cooling (e.g., Vertiv (VRT), Schneider Electric (SBGSF)), networking (e.g., Arista Networks (ANET)), and modular data centers (e.g., Equinix (EQIX)) are also poised to benefit as hyperscalers optimize infrastructure. Despite skepticism about AI CapEx durability, executives have reiterated the need for sustained investment as demand continues to outpace capacity, particularly for GPUs and custom accelerators. Investors should focus on these dynamics, recognizing that Big Tech’s AI investments are laying the foundation for long-term, multi-trillion-dollar opportunities across industries. Hence, investors should focus on companies with strong competitive moats, scalable business models, and diversified revenue streams. The following themes in the AI value chain offer significant potential.
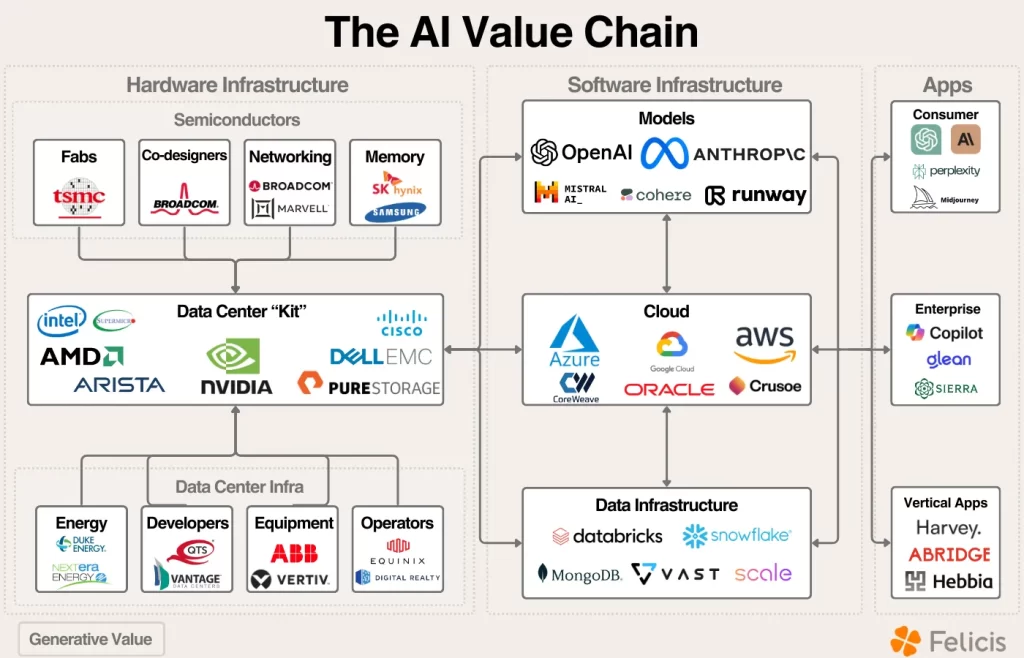
Investor Expectations and Timeline for Returns
On the other hand, investors are keeping a close watch on such CapEx investments and will be looking for pointers as to when these will finally bring real money home. For very large investments of this kind, this return- gestation period might have stretched over many years. First indicators of returns would come in after 2-3 years when the AI-enabled products and services start going into the market, and the revenues begin coming in.
To gauge the fruition of these investments, investors should look into:
- AI Service Revenue Growth: Success in the monetization of AI capabilities may be evident from revenue generated via AI-enabled services and products.
- Market Adoption Rates: The higher the adoption rates among consumers and enterprises, the better the scale at which AI solutions are being deployed.
- Operational Efficiency Metrics: Improvement in operational efficiencies and cost reductions due to the integration of AI highlight some of the internal benefits derived from investments in AI.
- Strategic Partnerships and Client Wins: New partnerships and client wins for AI services can be indicative of market confidence and commercial viability to offer AI.
While the current CapEx surge reflects a robust commitment to AI advancement, the timeline for realizing substantial returns may vary. Long-term investors would do well to take a big-picture perspective, recognizing that the potentially transformative power of AI will, like many other technological revolutions before it, take its sweet time to translate into significant financial returns.
The Foundations: The Data Center Market and Its Role in AI
The rise of generative AI and large-scale machine learning models has placed enormous demands on data centers, making them the linchpin of the AI revolution. The data center market today can be broken into three core pillars: compute, networking, and storage, with additional components such as energy infrastructure and cooling systems supporting these pillars. Hyperscalers like Amazon (AWS), Microsoft (Azure), and Google Cloud are leading massive CapEx cycles, building data centers optimized for AI workloads.
Jensen Huang of Nvidia once noted that half the cost of a modern data center goes into infrastructure—land, power, and cooling—while the other half supports the core technology, such as servers, networking switches, and GPUs. AI demands enormous compute resources for model training and inference, but the story doesn’t stop there. As AI applications scale, the bottlenecks extend beyond GPUs to areas like data flow optimization (networking) and power efficiency (cooling).
The scale of these data centers is astonishing. Nvidia DGX-based systems have become industry standards for training large AI models, combining GPUs, networking, and software. At the same time, data center operators like Equinix and Digital Realty (DLR) have emerged as critical enablers, providing colocation services and physical infrastructure.
What remains untapped is how edge data centers will grow to complement hyperscaler centers. Edge facilities, located closer to end users, will reduce latency and enable real-time AI inference for applications like autonomous vehicles, AR/VR, and IoT devices. Companies specializing in edge-specific cooling, networking, and micro-data centers are poised to capture this market.
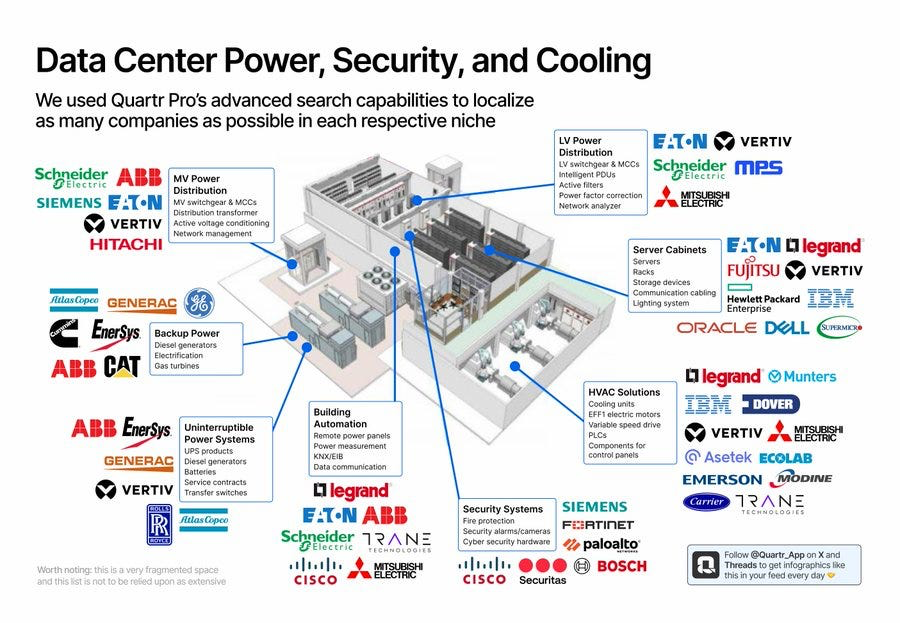
The Compute Market: Beyond Nvidia’s Dominance
At the heart of AI infrastructure lies the compute market, currently dominated by Nvidia GPUs. The company’s H100 GPUs set the gold standard for AI model training, achieving both performance and software integration through its CUDA ecosystem. Nvidia commands nearly 80% of the AI GPU market, generating over $14 billion in quarterly data center revenue. However, the compute market is beginning to fragment, offering openings for competitors.
AMD has emerged as Nvidia’s most credible challenger. Its MI300X GPUs provide better throughput at large batch sizes due to their larger VRAM capacity. While Nvidia’s software dominance keeps it ahead in real-world deployments, AMD’s price-to-performance ratio and increasing traction in inference workloads highlight its growing relevance.
Custom silicon is another force challenging the GPU monopoly. Hyperscalers like Google (TPUs), Amazon (Trainium), and Microsoft (Maia) have developed proprietary AI accelerators, targeting specific workloads and driving cost efficiencies. These ASICs (Application-Specific Integrated Circuits) offer a lower total cost of ownership for large-scale AI inference. Meta, too, has entered the fray with its MTIA chips, and startups like Cerebras, Groq, and d-Matrix are developing inference-optimized architectures to address AI’s growing deployment needs.
While GPUs dominate training, AI inference—the process of deploying trained models to generate predictions—presents a much broader market opportunity. Jensen Huang predicts that inference workloads will grow a billion times in volume over the coming decade. Inference differs from training in that it requires lower latency, distributed deployment, and on-demand scalability. This makes the market far more competitive, with AMD, Qualcomm (QCOM), Intel (INTC), and startups offering alternative solutions for edge and on-premise inference. The future of compute lies in specialization. Thus, investors should monitor startups developing ASICs for edge-specific workloads and companies advancing software optimization to improve hardware efficiency.
Networking: The Data Flow Bottleneck and Arista’s Advantage
AI compute advancements would be meaningless without robust data connectivity. As models grow in size and complexity, the movement of massive datasets between GPUs, servers, and storage systems has emerged as a key bottleneck. This has elevated networking to a mission-critical component within the AI infrastructure stack.
Nvidia’s acquisition of Mellanox in 2020 was a prescient move, positioning it as a leader in high-performance networking with its InfiniBand technology. InfiniBand offers lower latency and higher throughput than traditional Ethernet, making it ideal for AI workloads where rapid data flow between GPUs is critical. Nvidia’s integrated platforms, such as DGX SuperPods, combine InfiniBand and GPUs to deliver end-to-end AI systems, further entrenching its market leadership.
Meanwhile, Arista Networks has captured the Ethernet market for data centers, particularly among hyperscalers. Arista’s high-speed switches connect servers and storage systems, and CEO Jayshree Ullal predicts Ethernet will ultimately surpass InfiniBand as the preferred networking standard for AI. The rise of the Ultra Ethernet Consortium, aimed at developing Ethernet solutions for AI workloads, underscores this long-term trend.
For now, InfiniBand holds a dominant position in high-performance computing. However, investors should pay close attention to advancements in Ethernet and emerging startups offering new networking solutions. Companies like Marvell (MRVL) and Broadcom (AVGO), which design networking chips (NICs, DPUs), will play pivotal roles in optimizing data flow and reducing latency. The competition between InfiniBand and Ethernet remains unresolved, and the winner will shape the future of AI infrastructure.
AI Inference and Edge Computing: The Next Big Opportunity
While AI training has dominated the narrative so far, AI inference—where trained models are used to make predictions in real-world applications—represents the next growth frontier. Inference workloads are projected to far exceed training workloads, driven by the proliferation of AI applications across industries.
The economics of inference are fundamentally different. Unlike training, which relies on centralized clusters of GPUs, inference can occur closer to the end user, at the “edge.” This reduces latency and enables real-time decision-making for applications like self-driving cars, robotics, and smart devices. Companies are already building hardware optimized for edge inference, including Apple (Neural Engine), Qualcomm (Snapdragon NPUs), Intel (Gaudi chips), and AMD (Ryzen AI processors).
The competitive landscape for inference is far more fragmented than training. Nvidia still dominates this space, but its moat is smaller due to fewer requirements for system scalability and reliability. Startups like Groq, Cerebras, and Hailo are targeting niche markets with inference-optimized architectures that challenge Nvidia’s GPUs on cost and performance.
Another emerging trend is inference providers, such as Fireworks AI and Together AI, which abstract away the complexity of hardware management and offer APIs for running open-source models. This is akin to how cloud platforms revolutionized infrastructure provisioning. Companies like Coreweave and Crusoe, which provide GPU cloud infrastructure for inference, are also gaining traction.
Investors should focus on edge inference as a high-growth segment. The convergence of efficient small models, optimized edge hardware, and compelling use cases will create significant value. Companies developing inference-specific hardware, edge platforms, and energy-efficient systems will lead this transformation.
The Future Landscape and Investment Opportunities
The AI market is still in its early innings, and its value chain remains unevenly developed. The dominance of Nvidia in GPUs and Arista in connectivity highlights areas of maturity, but new opportunities are emerging across the ecosystem. Inference workloads are set to explode, and the market is far more open to competition than training. Companies offering inference-optimized hardware, such as ASICs and DPUs, and edge solutions will challenge incumbents and capture market share.
Networking, too, is ripe for disruption. While Nvidia’s InfiniBand leads today, Ethernet’s long-term potential and ongoing advancements cannot be ignored. Companies like Marvell, Broadcom, and startups working on AI-specific networking solutions will benefit as data center bottlenecks move beyond compute. The edge represents the most underexplored segment. As compute power moves closer to devices, opportunities will arise for hardware companies, edge data center operators, and software platforms enabling real-time AI applications.
Energy and cooling systems remain an overlooked but critical piece of the AI infrastructure puzzle. The power density of AI workloads requires innovations in energy management, efficient cooling, and grid optimization. Companies specializing in these areas, such as Vertiv and Schneider Electric, are well-positioned to grow alongside AI infrastructure demand.
In conclusion, investors should look beyond the obvious winners in GPUs and data center networking. The next phase of AI growth will be defined by specialization, efficiency, and decentralization. Compute will fragment, networking will evolve, and edge inference will scale. By focusing on these emerging opportunities, investors can position themselves at the forefront of the AI revolution.